
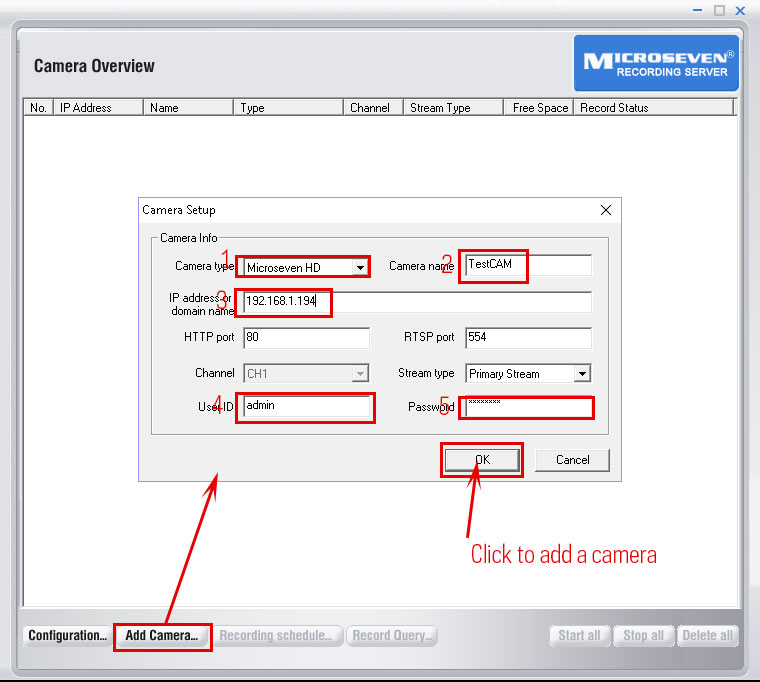
This stacking ensemble of deep learning method is a two-tier architecture, which includes an ensemble of base Multi-Layer Perception (MLP) classifiers and a fusion Support Vector Machine (SVM) classifier.
#Microseven delete selected videos android#
In such context, by studying the actions of malware, this work develops a novel framework SEDMDroid to detect Android malware, based on an enhanced stacking ensemble of deep learning algorithm. Malware poses a serious threat to user privacy, money, equipment and file integrity.
#Microseven delete selected videos software#
Due to its popularity and functionality, it is the main target of malicious software (malware). The openness and extensibility of Android have made it a popular platform for smart phones and a strong candidate to drive the Internet-of-Things. To the best of our knowledge, this paper is the first effort to shed light on the IP-spatial, temporal, entropy, and cloud service patterns of IoT devices in edge networks, and to explore these multidimensional behavioral fingerprints for IoT device classification, anomaly traffic detection, and network security monitoring for vulnerable and resource-constrained IoT devices on the Internet. As a first step towards understanding and mitigating diverse security threats of IoT devices, this paper develops an IoT traffic measurement framework on programmable and intelligent edge routers to automatically collect incoming, outgoing, and internal network traffic of IoT devices in edge networks, and to build multidimensional behavioral profiles which characterize who, when, what, and why on the behavioral patterns of IoT devices based on continuously collected traffic data. The recent spate of cyber attacks exploiting the vulnerabilities and insufficient security management of IoT devices have highlighted the urgency and challenges for securing billions of IoT devices and applications. Our experiments show that Rover achieves localization accuracies of 39.3 cm for the robot and 74.6 cm for the tags.Īs connected Internet-of-things (IoT) devices in smart homes, smart cities, and smart industries continue to grow in size and complexity, managing and securing them in distributed edge networks have become daunting but crucial tasks. We prototype Rover using off-the-shelf WiFi chips and customized backscatter tags. Our design addresses practical issues including interference among multiple tags, real-time processing, as well as the data marginalization problem in dealing with degenerated motions.
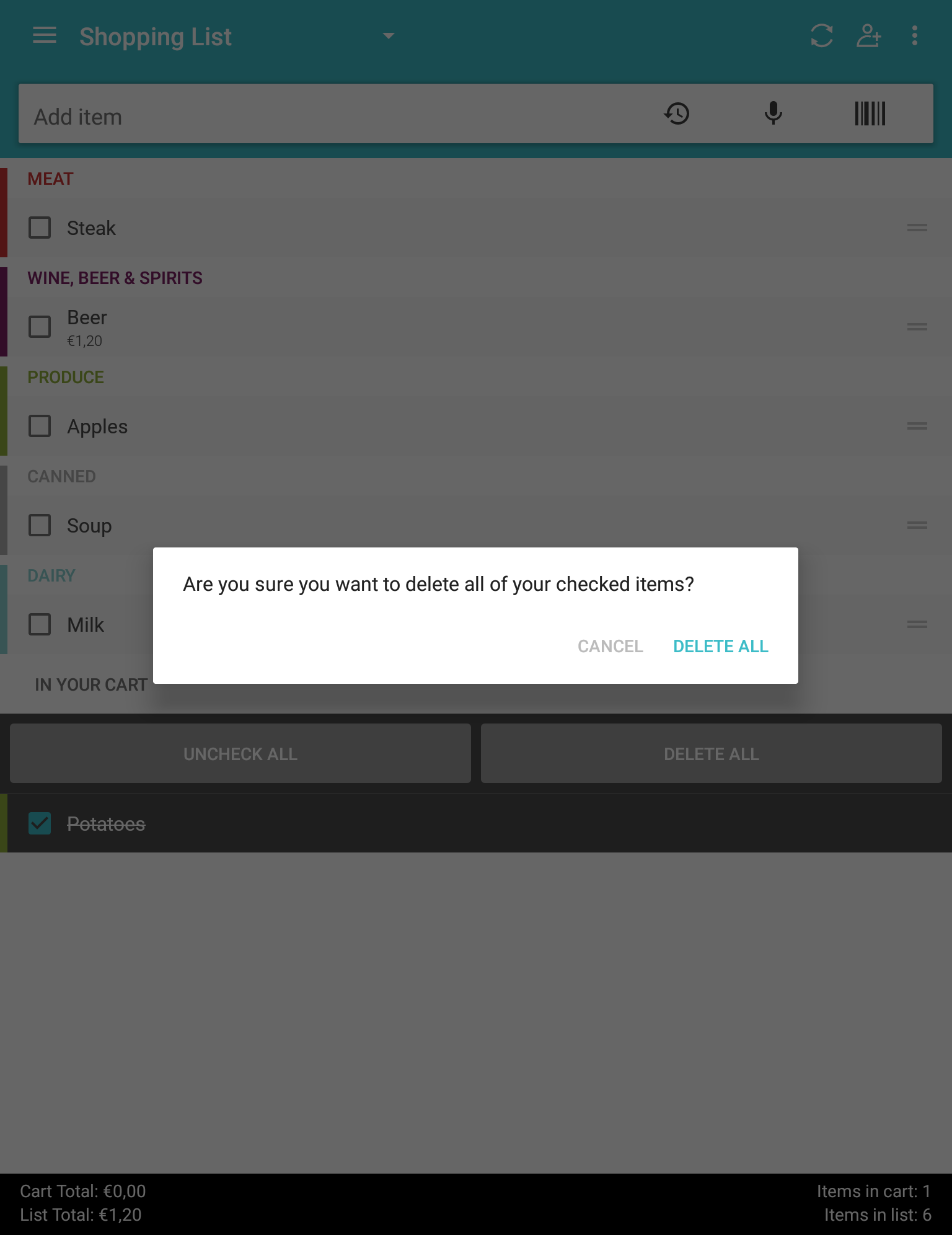
Rover runs in a joint optimization framework, fusing measurements from backscattered WiFi signals and inertial sensors to simultaneously estimate the locations of both the robot and the connected tags. To empower universal localization service, this paper presents Rover, an indoor localization system that localizes multiple backscatter tags without any start-up cost using a robot equipped with inertial sensors. However, current backscatter localization systems require prior knowledge of the site, either a map or landmarks with known positions, which is laborious for deployment. Localizing such backscatter tags is crucial for IoT-based smart applications. Recent years have witnessed the rapid proliferation of backscatter technologies that realize the ubiquitous and long-term connectivity to empower smart cities and smart homes. Using the network traffic of heterogeneous IoT devices collected at the router of a real-world smart home testbed and a public IoT dataset, we demonstrate that IoTAthena is able to characterize and generate activity signatures of IoT device activities and accurately unveil the sequence of IoT device activities from raw network traffic. Using sigMatch as a subfunction, actExtract can accurately unveil the sequence of various IoT device activities from the raw network traffic. For any given signature, sigMatch can capture all matches of the signature in the raw network traffic. IoTAthena has two novel polynomial time algorithms, sigMatch and actExtract. IoTAthena characterizes each IoT device activity using an activity signature consisting of an ordered sequence of IP packets with inter-packet time intervals. In this paper, we present a new system, named IoTAthena, to unveil IoT device activities from raw network traffic consisting of timestamped IP packets. The recent spate of cyber attacks towards Internet of Things (IoT) devices in smart homes calls for effective techniques to understand, characterize, and unveil IoT device activities.
